Our projects
AI to enable predictive maintenance in business aircraft
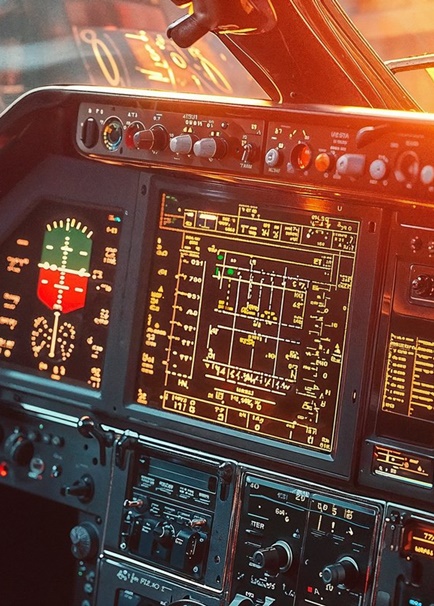
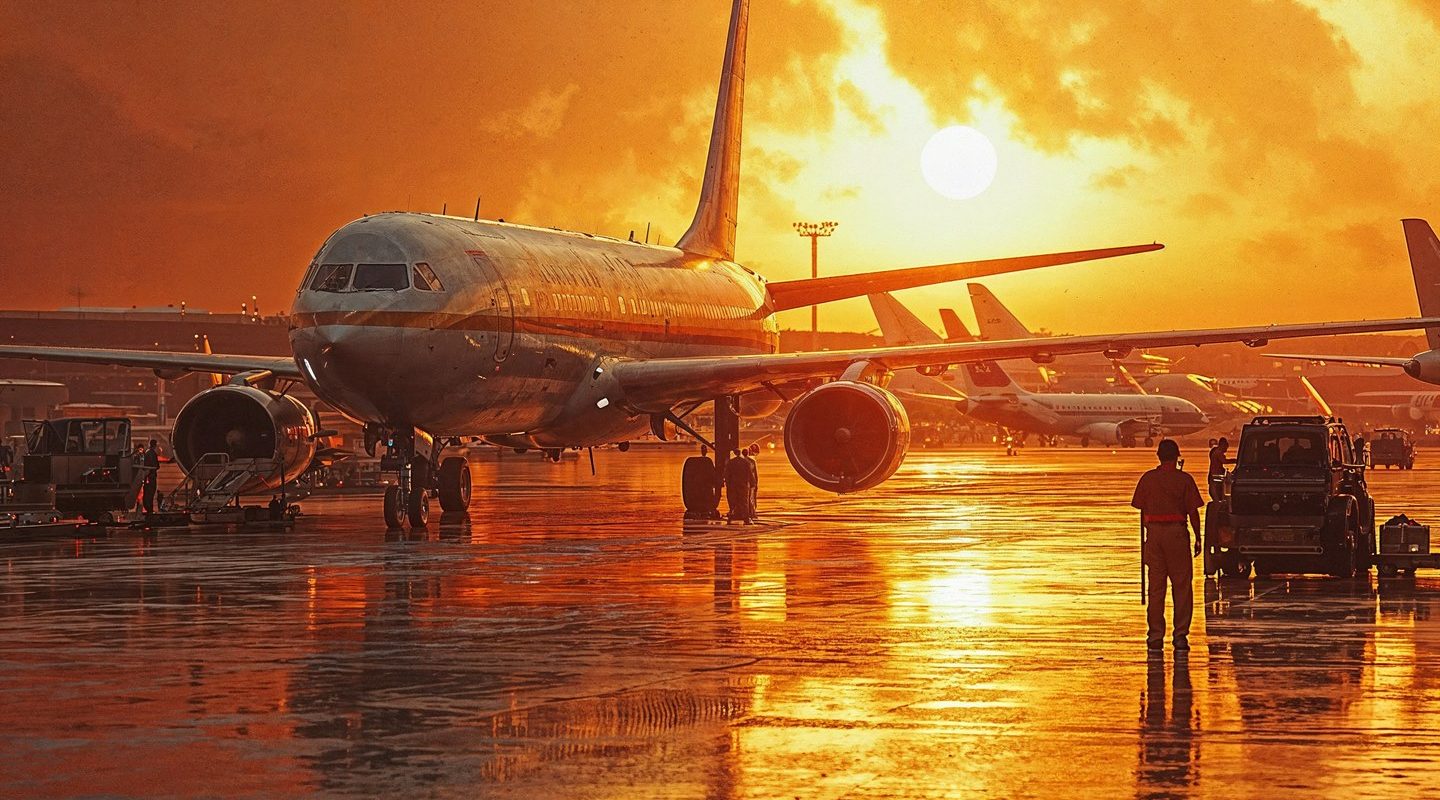
Reduce unplanned maintenance costs and prevent costly aircraft downtime by anticipating failures before they occur.
IVADO Labs developed an advanced AI solution that analyzes equipment conditions in real time, diagnoses anomalies, and predicts future failures for proactive management.
The AI solution has improved aircraft reliability by reducing downtime and optimizing component life, covering two-thirds of critical maintenance scenarios.
The first step involves continuous monitoring of the condition of various components to identify signs of potential issues before they worsen. “It’s somewhat like the ‘check engine’ light on a car,” explains Ashwin Rai, Senior Data Scientist at IVADO Labs.
While condition monitoring can detect an anomaly, it cannot alone determine the root cause. However, it serves as the critical starting point in the predictive maintenance process.
To identify an anomaly, it’s important to first understand the normal behavior of the aircraft. “We had access to historical flight data from the aircraft manufacturer, which allowed us to deploy machine learning models to understand the expected behavior during different stages of flight, such as takeoff and landing,” explains Rai.]
Once normal behavior is established, any deviation from this baseline can indicate a potential issue.
“By analyzing hundreds of flights, we can precisely determine the normal brake temperature during landing. If this braking temperature is reached while cruising at 30,000 feet, there’s an issue,” illustrates Morgan Bye, Senior Team Lead at IVADO Labs.
This applies equally to more subtle deviations. For instance, a slightly higher or lower than normal temperature during landing could indicate an underlying problem.
Beyond temperature readings, a wide range of other parameters are monitored, including pressure, humidity, and electrical currents. Even seemingly secondary components like an in-cabin espresso machine are monitored, since they also require maintenance in the event of a malfunction.
The challenge lies in distinguishing actual issues from false alarms. “Abnormal behavior might be acceptable and simply triggered by pilot maneuvering or variable flight conditions,” notes Bye.
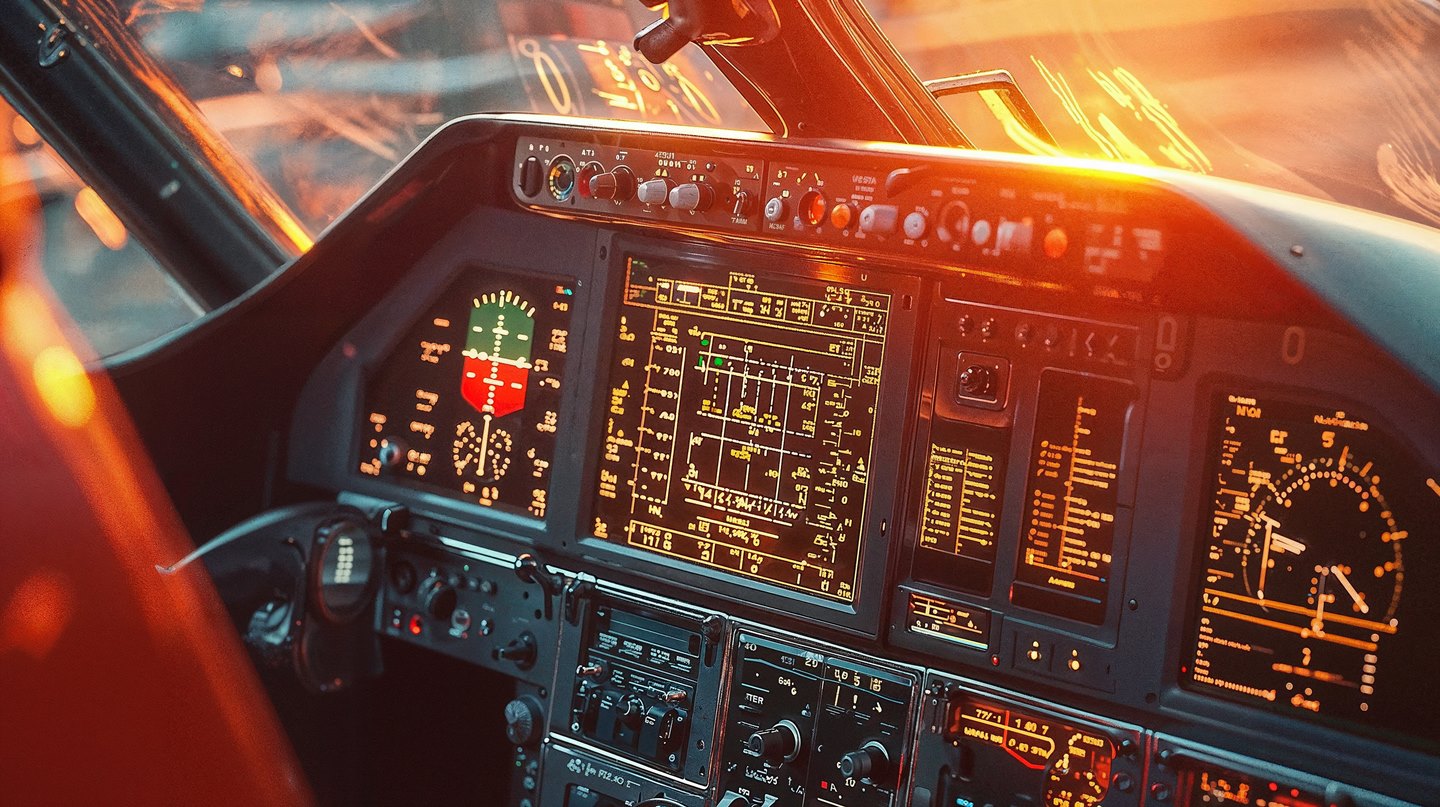
Once a potential issue is detected, the next step is to diagnose its cause. This involves understanding the nature of the anomaly and enabling the maintenance team to select the appropriate corrective actions from the available options. The diagnostic process transforms raw sensor data into actionable information, guiding maintenance teams in their repair efforts.
The diagnostic system developed by IVADO Labs combines AI models with engineering expertise. “We used a hybrid approach, integrating data-driven AI methods with the expertise of the company’s engineers,” says Rai. “This results in a more powerful system that blends human expertise with AI capabilities.”
Collaborating with the aircraft manufacturer also helped prioritize which systems should be analyzed using artificial intelligence. “We asked which issues were the hardest to diagnose and which ones most often grounded the aircraft. For example, tires can easily be inspected by a ground crew, whereas a fuel pump located inside the engine may require dismantling, which is much more complex. So we focused our efforts on the most strategic components, particularly those that were not adequately addressed by existing procedures,” explains Bye.
IVADO Labs’ diagnostic approach provides a deeper understanding of the equipment. AI models can identify data trends that may be difficult for even human experts to detect, providing valuable insights.
For instance, if the brakes are overheating, the system can analyze data from related components like hydraulic systems. This helps determine whether the issue originates from the braking mechanism itself or from another connected system.
One of the biggest challenges was to make the AI-generated insights understandable and actionable for maintenance teams. AI can identify complex patterns and correlations, but these need to be translated into practical recommendations. “We worked closely with the engineers to ensure the system’s outputs were both accurate and useful,” notes Bye.
By the end of the project, which lasted less than two years, the AI solution developed by IVADO Labs was able to provide useful diagnostics on 11 subsystems of the aircraft, addressing two-thirds of the most common scenarios that ground aircraft.
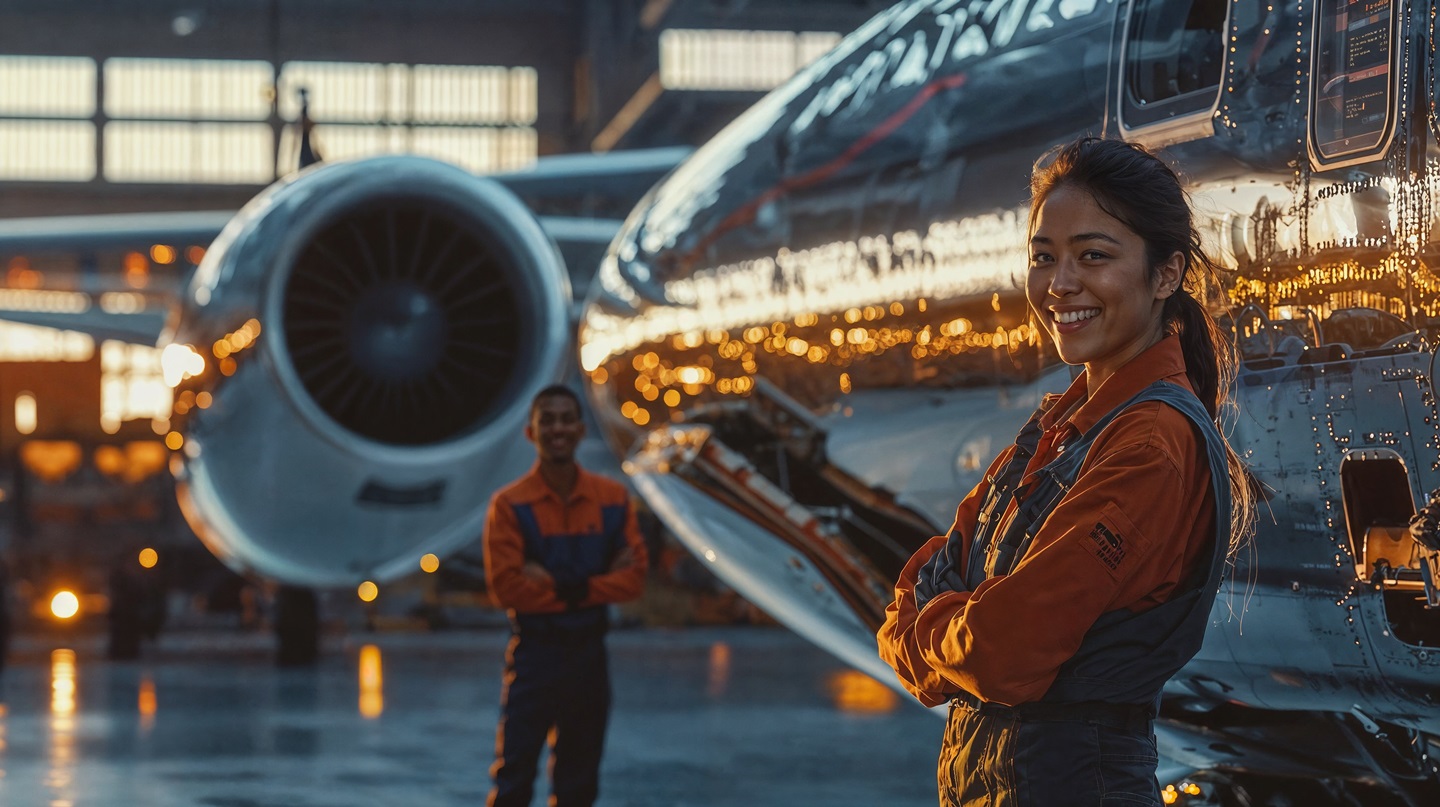
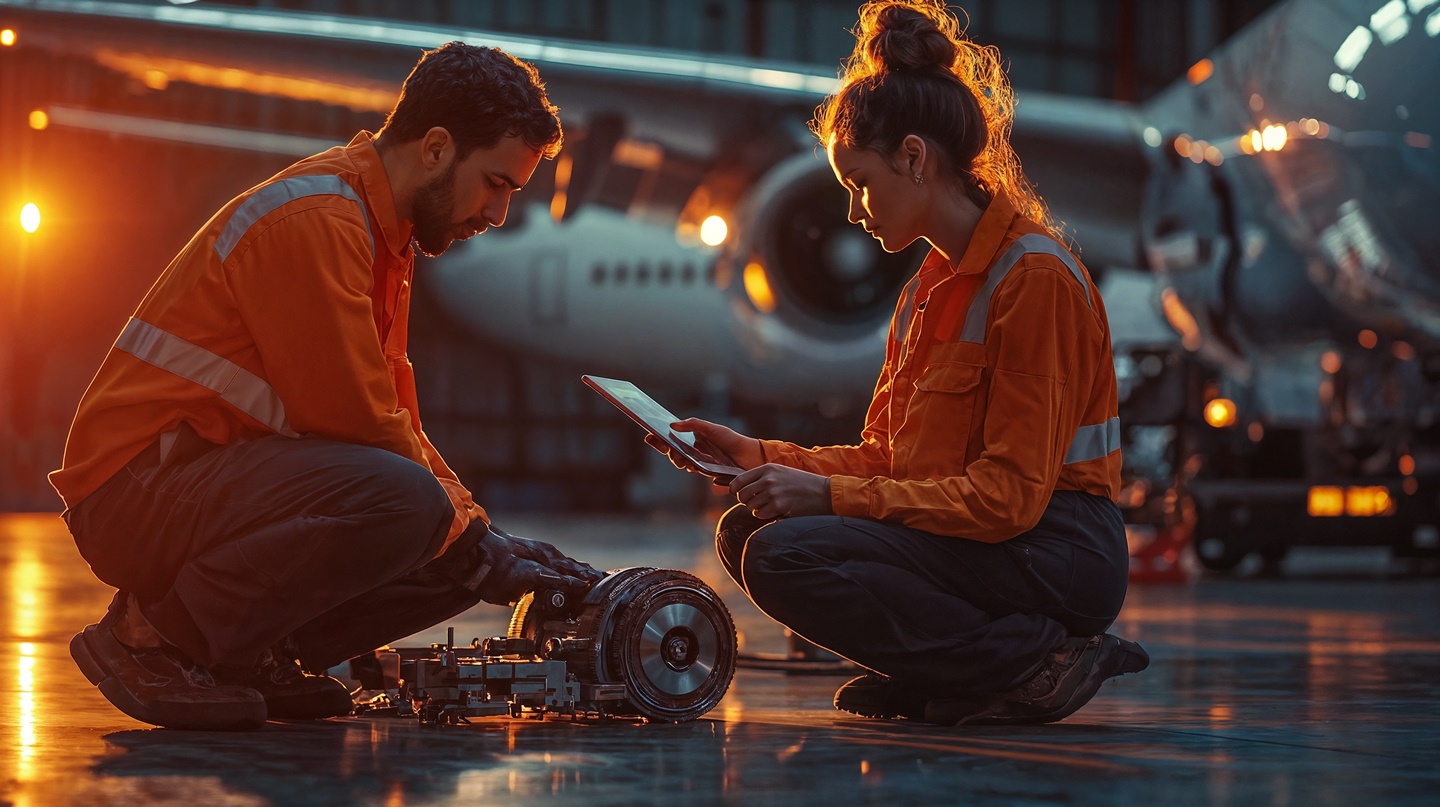
Prognostics are a key goal of predictive maintenance. They involve predicting when a component is likely to fail, enabling just-in-time maintenance, reducing downtime, and maximizing the lifespan of parts. Since it requires predicting future events based on current and historical data, it’s a complex approach.
In the case of business aircraft, IVADO Labs combined AI and traditional reliability engineering methods to develop a prognostic model. The model can predict when a component is likely to fail based on its current condition, historical data, and information provided by the manufacturer.
“The model we deployed is well known by the manufacturer’s engineers. We adapted it to work with our AI systems for condition monitoring, allowing us to produce performance and reliability forecasts of up to 10 years for each component,” explains Rai.
“If we use the engine check light analogy again, the goal is to replace it with a traffic light system for each part of the aircraft: green shows that everything is fine, amber means it should be checked during the next service, and red indicates that it requires immediate attention,” adds Morgan Bye.
As more data is collected from business aircraft equipped with predictive maintenance systems, the accuracy and efficiency of machine learning models will continue to improve, delivering even greater value to clients.
“We’ve established a solid foundation to enable continuous improvement as new data becomes available,” says Soufiane El Mdari, Senior Product Manager at IVADO Labs.