Your challenges > Managing inventory
AI for inventory management
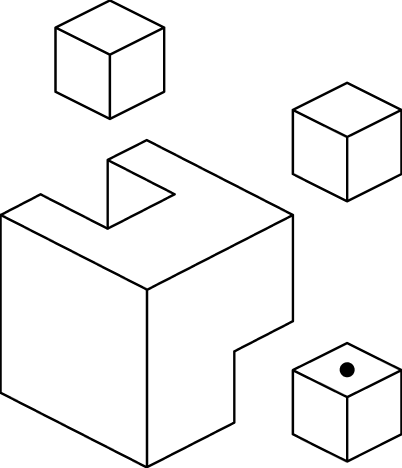
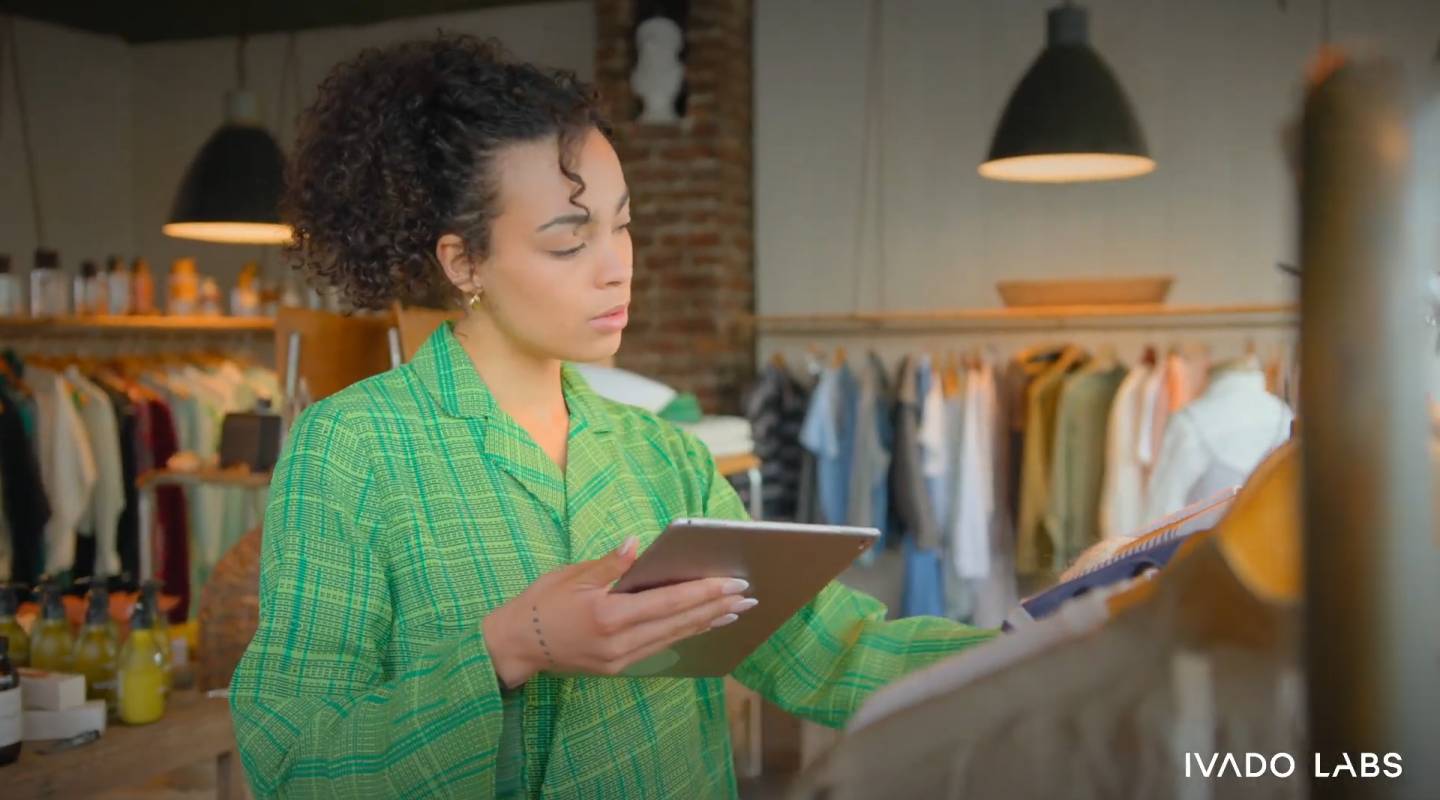
Artificial intelligence can enhance inventory management by augmenting efficiency, accuracy and predictive capabilities into business processes—while optimizing trade-offs between cost and availability.
AI’s ability to process and learn from extensive and varied datasets at scale can help attain much more precise and granular forecasts. The resulting insights into future demand across an organization’s products and locations can inform management and fulfillment policies. These policies can be optimized by AI, accounting for costs, uncertainty in forecasts and lead time, as well as the complexity of global, multi-echelon distribution networks. This ensures service levels can be maintained or improved, while reducing stock requirements and emergency shipments.
main challenges
Demand and lead time uncertainty have been amplified due to recent disruptions in global supply chains. This results in challenges when determining the right target service level—one that strikes a balance between sales opportunities and inventory costs. In this context, decision-makers are faced with three key challenges: demand forecasting, inventory optimization and fulfilment optimization.
Level up your inventory management with
AI benefits!
Streamlining back-office and communication processes
Optimizing fulfillment decisions for sales
Optimizing inventory positioning across a multi-echelon network
Optimizing inventory targets to reduce overhead while meeting demand
Conducting what-if analyses to support tactical and strategic decisions
Profitability hinges on accurate demand forecasting, and that’s where artificial intelligence can help: by learning from historical sales patterns, market trends, customer demographics, and other inputs, AI can provide more accurate demand prediction in fluctuating environments.
This enhanced forecasting can inform inventory management decisions, helping businesses better understand what to replenish and how often. Indeed, optimizing inventory levels to manage uncertainty against sales targets and service levels while balancing supply chain costs allows decision-makers to make proactive procurement decisions. AI can also mitigate risk by flagging potential issues such as stockouts, overstocking and delays.
In the supply chain, AI can optimize order fulfillment, such as determining which distribution centers can deliver the fastest and most cost-effectively—taking into account factors such as shipping costs, delivery timelines and forecasted demand—to prevent delays and boost customer satisfaction.
A typical market approach to inventory management involves optimizing each location individually, propagating demand through the network using static lead times—while ignoring uncertainty—and providing limited explainability of inventory drivers.
IVADO Labs’ approach to multi-echelon network optimization is more holistic. It optimizes the distribution network as a whole, propagates demand through the network by accounting for lead time uncertainty, and assesses the impact of inventory drivers.
The use of AI in demand forecasting can significantly increase accuracy, allowing decision-makers to make informed decisions about inventory management, pricing strategies, future trends and more.
AI can optimize the supply chain in a number of ways, including efficiency, resilience and cost-effectiveness. In the manufacturing sector, AI in the supply chain can help determine when to purchase raw materials, in which quantity and at what price. Additionally, AI can help manage purchase orders dynamically to adapt to changes in production plans.
AI can help ensure you have the right product, in the right place, at the right time for customers. This not only helps avoid lost sales, but also improves service levels, minimizes disruptions in the supply chain, and enhances planning for store and e-commerce space and promotions. This contributes to higher inventory accuracy, lower holding costs and, ultimately, better customer satisfaction.
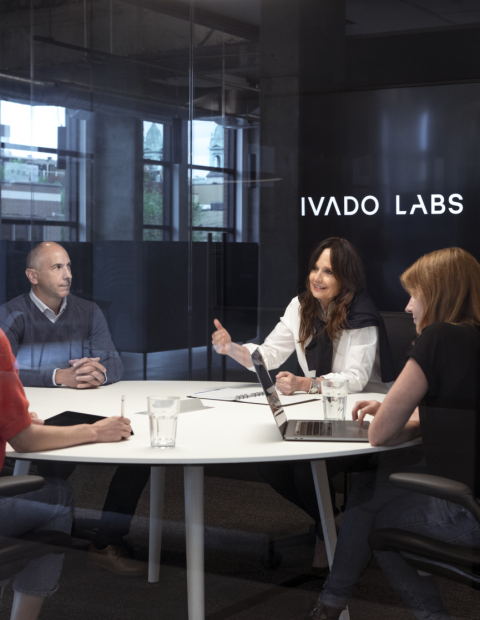